manifold learning and dimensionality reduction
with H. Lee, J. Perea, N. Schonscheck, and M. Weinstein
Stiefel manifolds, consisting of n by k matrices with orthonormal columns, often arise in the context of signal and image processing, machine learning, and numerical optimization. In this project we construct a dimensional reduction scheme for data on Stiefel manifolds which finds an embedded lower-dimensional Stiefel manifold approximating the given data. Importantly, the projection to this lower-dimensional manifold is O(k)-equivariant.
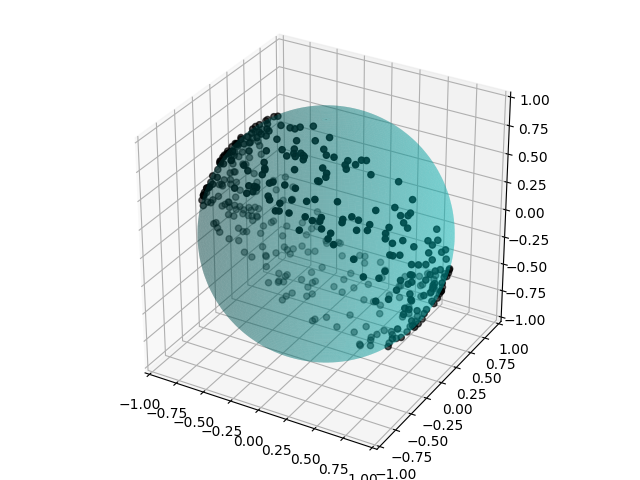
A point cloud on the 2-sphere St(3,1).